These hyper-realistic mannequins are designed to facilitate image quality evaluation, each having a specified spectral response for skin tone and all the details of a real human face.
Due to the static nature of these mannequins (compared to a real person who moves), test scenes using these mannequins are absolutely identical, making resulting measurements repeatable and reliable. Further, using a mannequin can save precious testing time for a lab operator. We have three models of mannequin, each with a different skin tone. We test and measure each realistic head in our labs before shipping to ensure the same quality, and assign each mannequin a unique identification number to facilitate high traceability.
General specifications:
- Material: Polyurethane foam, proprietary skin-like pigmentation, silicon, real hair, glass eyes
- 3 standard skin-tones available (custom on request)
- Support trolley: SUPPORT_REALMAN
- Motorized rotation system: ROT_REALMAN_001
AI measurements on Realistic Mannequins
Each mannequin is a highly-repeatable hyper-realistic stand-in for a human model, accurate down to the finest details of their features and the spectral response of their skin. These mannequins can be used by themselves or integrated in more complex setups.
The resulting images can be evaluated perceptually for many image quality attributes, and the high degree of repeatability also allows objective measurements, such as the exposure on the face, and AI-based metrics, such as the preservation of details. The preservation of detail metric focuses on specific details of each mannequin. For Eugene, the details are best appreciated on his thick beard.
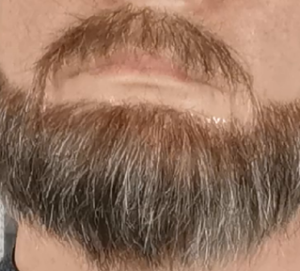
Eugene’s ROI of Detail Preservation Metric
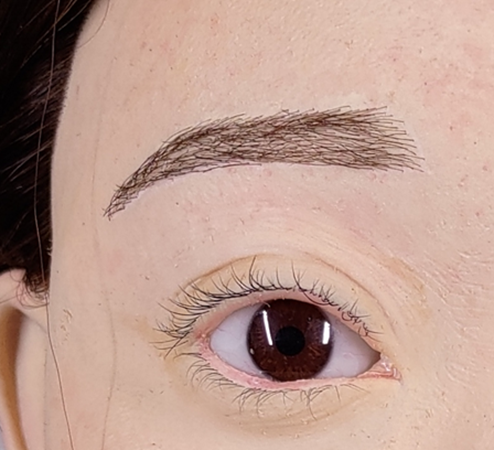
Sienna’s ROI of Detail Preservation Metric
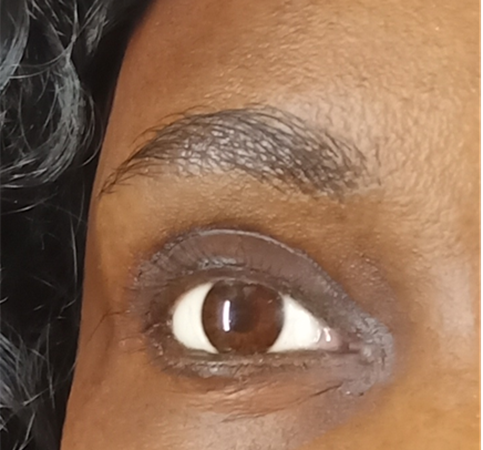
Diana’s ROI of Detail Preservation Metric
Our automatic metric relies on a convolutional neural network (CNN) that reproduces in less than a second detail preservation perceptual analysis of a quality expert through their human visual system. The results are provided in a perceptual scale quantified in just-objectionable differences, or JOD, which has a very straight-forward interpretation: a difference of one unit in the metric results of two images means that 75% of the observers will be able to see a difference in the level of detail of the two images. These tools can integrate or replace perceptual analysis thus speeding up considerably the time to complete an evaluation.